What is Design of Experiments DOE?
Table Of Content

They are done by scientists and engineers, among others, in order to understand which inputs have a major impact on output and what input levels should be targeted to reach a desired outcome (output). Simply put, DoE is a way to collect information during the experiment and then determine what factors or which processes could lead to the desired result. Instead of concepts like power and aliasing, which are relevant to determining which effects are real, RSM uses concepts relating to the errors when making predictions in the relevant design space. Fractional factorial designs assume that while there may be many effects, only a few are important.
School's out... and so is OFAT (one-factor-at-a-time) experimentation.
You need to have a deliberate process to eliminate potential biases from the conclusions, and random assignment is a critical step. If you have a treatment group and a control group then, in this case, you probably only have one factor with two levels. Run all possible combinations of factor levels, in random order to average out effects of lurking variables. A more effective and efficient approach to experimentation is to use statistically designed experiments (DOE).
Hypothesis Testing
As you can already tell, OFAT is a more structured approach compared to trial and error. Run the second experiment by varying time, to find the optimal value of time (between 4 and 24 hours). Change the value of the one factor, then measure the response, repeat the process with another factor.
Identify the main effects of your factors
Emergence is one reason biologists often lack well-developed, robust theoretical frameworks to guide their experiments. You can visualize, explore your model and find the most desirable settings for your factors using the JMP Prediction Profiler. Notice that none of them has trials conducted at a low temperature and time AND near optimum conditions. Experiments are likely to be carried out via trial and error or one-factor-at-a-time (OFAT) method. Plus, we will we have support for different types of regression models. For example, we can estimate what we call a linear model, or an interaction model, or a quadratic model.
3 - Steps for Planning, Conducting and Analyzing an Experiment
By eliminating potential bias, randomization safeguards the truthfulness of the experimental outcomes, making the findings generalizable and credible. The ethical considerations in research design form the bedrock of DoE. They are the safeguards that ensure research not only advances knowledge but does so with respect for the subjects involved, the data collected, and the ecosystems within which research is conducted. These considerations demand transparency, consent, and honesty, upholding the values of respect and dignity in every phase of the experimental process.
Optimize the Important Factors/Variables
They can also help you identify when technical restrictions apply and avoid making the wrong choice. Instead of trying to fit your DOE campaign into a single experiment, think of the DOE process as a collection of sub-experiments. And for each sub-experiment, you might use a different design—as every type of DOE design at each step is intimately linked to a phase in the DOE campaign. They can move you rapidly from your initial “thought experiment” to optimized conditions and robust data. There’s also a whole other way of doing designs, where you use software to create a bespoke design to your exact requirements. These are called optimal designs, and it’s a topic for another day.
Blocking is a technique to include other factors in our experiment which contribute to undesirable variation. Much of the focus in this class will be to creatively use various blocking techniques to control sources of variation that will reduce error variance. For example, in human studies, the gender of the subjects is often an important factor.
Introduction to Design of Experiments
This will provide information as to potentially significant factors without consuming your whole budget. Once you’ve identified the best potential factors, you can do a full factorial with the reduced number of factors. A full factorial design provides information about all the possible interactions.
We would have missed out acquiring the optimal temperature and time settings based on our previous OFAT experiments. DOE applies to many different investigation objectives, but can be especially important early on in a screening investigation to help you determine what the most important factors are. Then, it may help you optimize and better understand how the most important factors that you can regulate influence the responses or critical quality attributes.
Experimental design and multiple response optimization. Using the desirability function in analytical methods ... - ScienceDirect.com
Experimental design and multiple response optimization. Using the desirability function in analytical methods ....
Posted: Sun, 15 Jun 2014 07:00:00 GMT [source]
At any stage in your DOE campaign, you could take your pick from several designs, depending on your assumptions, goals, available run numbers, and so on. The number of possible designs on offer can sometimes seem a bit overwhelming. The variance of the estimate X1 of θ1 is σ2 if we use the first experiment. But if we use the second experiment, the variance of the estimate given above is σ2/8. Thus the second experiment gives us 8 times as much precision for the estimate of a single item, and estimates all items simultaneously, with the same precision. What the second experiment achieves with eight would require 64 weighings if the items are weighed separately.
This evolved in the 1960s when medical advances were previously based on anecdotal data; a doctor would examine six patients and from this wrote a paper and published it. The incredible biases resulting from these kinds of anecdotal studies became known. The outcome was a move toward making the randomized double-blind clinical trial the gold standard for approval of any new product, medical device, or procedure. The scientific application of the statistical procedures became very important.
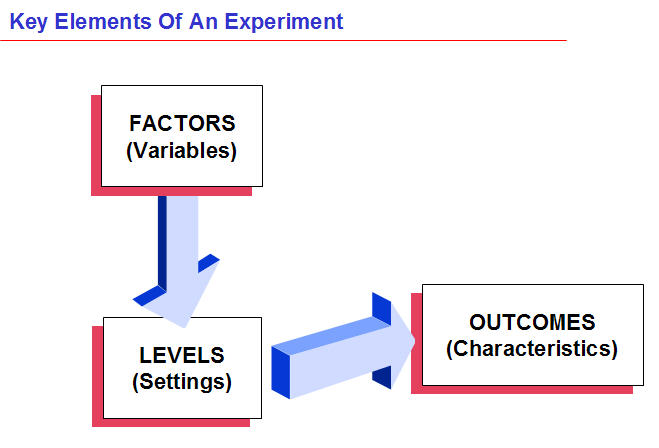
Design of Experiments (DOE) offers a daunting compilation of types of design. As a beginner, understanding which one is right for your needs can feel like an impossible task. In 1950, Gertrude Mary Cox and William Gemmell Cochran published the book Experimental Designs, which became the major reference work on the design of experiments for statisticians for years afterwards. Around 1990 Six Sigma, a new way of representing CQI, became popular. Now it is a company and they employ a technique which has been adopted by many of the large manufacturing companies. This is a technique that uses statistics to make decisions based on quality and feedback loops.
After viewing them, the customer then ranked the different mockups from most preferred to least preferred. The ranking provided the numerical value of that combination. To keep matters simple, they went with a quarter fraction design, or 16 different mockups. Otherwise, you’re asking customers to try and differentiate their preference and rank way too many options. Your process variables have different impacts on your output. We usually talk about "treatment" factors, which are the factors of primary interest to you.
DOE also identifies and explores the interactions between those factors. This allows us to optimize the performance and robustness of our processes or assays. This is an essential component of any experiment that is going to have validity. If you are doing a comparative experiment where you have two treatments, a treatment and a control, for instance, you need to include in your experimental process the assignment of those treatments by some random process.
So the selected experimental plan will support a specific type of model. Remember to let your DOE campaign stage guide your design choices. It can also be helpful to think about how much work you can actually do—and find a design that fits your budget. Typically, if you have an unmanageable number of runs, you’ve likely picked the wrong design. It’s easy to get overwhelmed by all the design choices that most DOE software gives you. Most DOE software, especially our own, is also there to help guide you.
Kishen in 1940 at the Indian Statistical Institute, but remained little known until the Plackett–Burman designs were published in Biometrika in 1946. R. Rao introduced the concepts of orthogonal arrays as experimental designs. This concept played a central role in the development of Taguchi methods by Genichi Taguchi, which took place during his visit to Indian Statistical Institute in early 1950s. His methods were successfully applied and adopted by Japanese and Indian industries and subsequently were also embraced by US industry albeit with some reservations. It is best that a process be in reasonable statistical control prior to conducting designed experiments.
Comments
Post a Comment